COVID-19
Zoom is Now Worth More Than the World’s 7 Biggest Airlines
Zoom Is Now Worth More Than The 7 Biggest Airlines
Amid the COVID-19 pandemic, many people have transitioned to working—and socializing—from home. If these trends become the new normal, certain companies may be in for a big payoff.
Popular video conferencing company, Zoom Communications, is a prime example of an organization benefiting from this transition. Today’s graphic, inspired by Lennart Dobravsky at Lufthansa Innovation Hub, is a dramatic look at how much Zoom’s valuation has shot up during this unusual period in history.
The Zoom Boom, in Perspective
As of May 15, 2020, Zoom’s market capitalization has skyrocketed to $48.8 billion, despite posting revenues of only $623 million over the past year.
What separates Zoom from its competition, and what’s led to the app’s massive surge in mainstream business culture?
Industry analysts say that business users have been drawn to the app because of its easy-to-use interface and user experience, as well as the ability to support up to 100 participants at a time. The app has also blown up among educators for use in online learning, after CEO Eric Yuan took extra steps to ensure K-12 schools could use the platform for free.
Zoom meeting participants have skyrocketed in past months, going from 10 million in December 2019 to a whopping 300 million as of April 2020.
The Airline Decline
The airline industry has been on the opposite end of fortune, suffering an unprecedented plummet in demand as international restrictions have shuttered airports:
The world’s top airlines by revenue have fallen in total value by 62% since the end of January:
Airline | Market Cap Jan 31, 2020 | Market Cap May 15, 2020 |
---|---|---|
Southwest Airlines | $28.440B | $14.04B |
Delta | $35.680B | $12.30B |
United | $18.790B | $5.867B |
International Airlines Group | $14.760B | $4.111B |
Lufthansa | $7.460B | $3.873B |
American | $11.490B | $3.886B |
Air France | $4.681B | $2.137B |
Total Market Cap | $121.301B | $46.214B |
Source: YCharts. All market capitalizations listed as of May 15, 2020.
With countries scrambling to contain the spread of COVID-19, many airlines have cut travel capacity, laid off workers, and chopped executive pay to try and stay afloat.
If and when regular air travel will return remains a major question mark, and even patient investors such as Warren Buffett have pulled out from airline stocks.
Airline | % Change in Total Returns (Jan 31-May 15, 2020) |
---|---|
United | -72.91% |
International Airlines Group | -72.16% |
American | -65.76% |
Delta | -65.39% |
Air France | -54.34% |
Southwest Airlines | -56.35% |
Lufthansa | -48.08% |
Source: YCharts, as of May 15, 2020.
The world has changed for the airlines. The future is much less clear to me about how the business will turn out.
—Warren Buffett
What Does the Future Hold?
Zoom’s recent success is a product of its circumstances, but will it last? That’s a question on the mind of many investors and pundits ahead of the company’s Q1 results to be released in June.
It hasn’t been all smooth-sailing for the company—a spate of “Zoom Bombing” incidents, where uninvited people hijacked meetings, brought the app’s security measures under scrutiny. However, the company remained resilient, swiftly providing support to combat the problem.
Meanwhile, as many parts of the world begin taking measures to restart economic activity, airlines could see a cautious return to the skies—although any such recovery will surely be a “slow, long ascent”.
Correction: Changed the graphics to reflect 300 million daily active “meeting participants” as opposed to daily active users.
Healthcare
Visualizing How COVID-19 Antiviral Pills and Vaccines Work at the Cellular Level
Despite tackling the same disease, vaccines and antiviral pills work differently to combat COVID-19. We visualize how they work in the body.
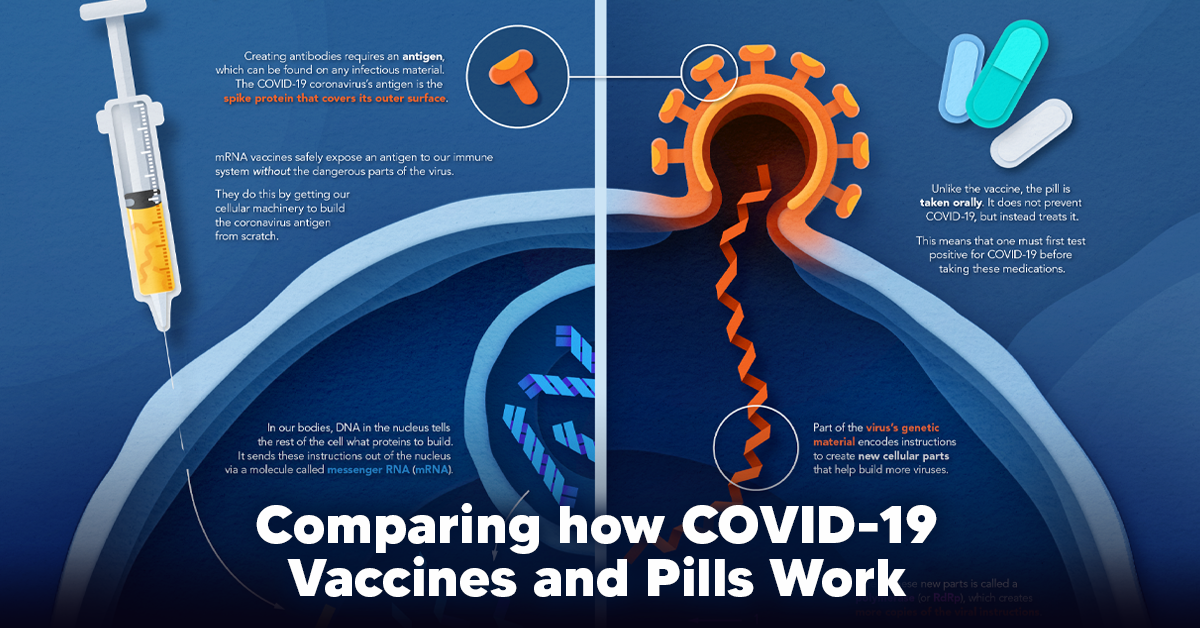
Current Strategies to Tackle COVID-19
Since the pandemic started in 2020, a number of therapies have been developed to combat COVID-19.
The leading options for preventing infection include social distancing, mask-wearing, and vaccination. They are still recommended during the upsurge of the coronavirus’s latest mutation, the Omicron variant.
But in December 2021, The United States Food and Drug Administration (USDA) granted Emergency Use Authorization to two experimental pills for the treatment of new COVID-19 cases.
These medications, one made by Pfizer and the other by Merck & Co., hope to contribute to the fight against the coronavirus and its variants. Alongside vaccinations, they may help to curb extreme cases of COVID-19 by reducing the need for hospitalization.
Despite tackling the same disease, vaccines and pills work differently:
Vaccines | Pills |
---|---|
Taken by injection | Taken by mouth |
Used for prevention | Used for treatment only |
Create an enhanced immune system by stimulating antibody production | Disrupt the assembly of new viral particles |
How a Vaccine Helps Prevent COVID-19
The main purpose of a vaccine is to prewarn the body of a potential COVID-19 infection by creating antibodies that target and destroy the coronavirus.
In order to do this, the immune system needs an antigen.
It’s difficult to do this risk-free since all antigens exist directly on a virus. Luckily, vaccines safely expose antigens to our immune systems without the dangerous parts of the virus.
In the case of COVID-19, the coronavirus’s antigen is the spike protein that covers its outer surface. Vaccines inject antigen-building instructions* and use our own cellular machinery to build the coronavirus antigen from scratch.
When exposed to the spike protein, the immune system begins to assemble antigen-specific antibodies. These antibodies wait for the opportunity to attack the real spike protein when a coronavirus enters the body. Since antibodies decrease over time, booster immunizations help to maintain a strong line of defense.
*While different vaccine technologies exist, they all do a similar thing: introduce an antigen and build a stronger immune system.
How COVID Antiviral Pills Work
Antiviral pills, unlike vaccines, are not a preventative strategy. Instead, they treat an infected individual experiencing symptoms from the virus.
Two drugs are now entering the market. Merck & Co.’s Lagevrio®, composed of one molecule, and Pfizer’s Paxlovid®, composed of two.
These medications disrupt specific processes in the viral assembly line to choke the virus’s ability to replicate.
The Mechanism of Molnupiravir
RNA-dependent RNA Polymerase (RdRp) is a cellular component that works similar to a photocopying machine for the virus’s genetic instructions. An infected host cell is forced to produce RdRp, which starts generating more copies of the virus’s RNA.
Molnupiravir, developed by Merck & Co., is a polymerase inhibitor. It inserts itself into the viral instructions that RdRp is copying, jumbling the contents. The RdRp then produces junk.
The Mechanism of Nirmatrelvir + Ritonavir
A replicating virus makes proteins necessary for its survival in a large, clumped mass called a polyprotein. A cellular component called a protease cuts a virus’s polyprotein into smaller, workable pieces.
Pfizer’s antiviral medication is a protease inhibitor made of two pills:
- The first pill, nirmatrelvir, stops protease from cutting viral products into smaller pieces.
- The second pill, ritonavir, protects nirmatrelvir from destruction by the body and allows it to keep working.
With a faulty polymerase or a large, unusable polyprotein, antiviral medications make it difficult for the coronavirus to replicate. If treated early enough, they can lessen the virus’s impact on the body.
The Future of COVID Antiviral Pills and Medications
Antiviral medications seem to have a bright future ahead of them.
COVID-19 antivirals are based on early research done on coronaviruses from the 2002-04 SARS-CoV and the 2012 MERS-CoV outbreaks. Current breakthroughs in this technology may pave the way for better pharmaceuticals in the future.
One half of Pfizer’s medication, ritonavir, currently treats many other viruses including HIV/AIDS.
Gilead Science is currently developing oral derivatives of remdesivir, another polymerase inhibitor currently only offered to inpatients in the United States.
More coronavirus antivirals are currently in the pipeline, offering a glimpse of control on the looming presence of COVID-19.
Author’s Note: The medical information in this article is an information resource only, and is not to be used or relied on for any diagnostic or treatment purposes. Please talk to your doctor before undergoing any treatment for COVID-19. If you become sick and believe you may have symptoms of COVID-19, please follow the CDC guidelines.
-
Debt1 week ago
How Debt-to-GDP Ratios Have Changed Since 2000
-
Markets2 weeks ago
Ranked: The World’s Top Flight Routes, by Revenue
-
Countries2 weeks ago
Population Projections: The World’s 6 Largest Countries in 2075
-
Markets2 weeks ago
The Top 10 States by Real GDP Growth in 2023
-
Demographics2 weeks ago
The Smallest Gender Wage Gaps in OECD Countries
-
United States2 weeks ago
Where U.S. Inflation Hit the Hardest in March 2024
-
Green2 weeks ago
Top Countries By Forest Growth Since 2001
-
United States2 weeks ago
Ranked: The Largest U.S. Corporations by Number of Employees