Technology
Infographic: The Future of Food
The Future of Food: How Tech Is Changing Our Food Systems
The urban population is exploding around the globe, and yesterday’s food systems will soon be sub-optimal for many of the megacities swelling with tens of millions of people.
Further, issues like wasted food, poor working conditions, polluted ecosystems, mistreated animals, and greenhouse gases are just some of the concerns that people have about our current supply chains.
Today’s infographic from Futurism shows how food systems are evolving – and that the future of food depends on technologies that enable us to get more food out of fewer resources.
The Next Gen of Food Systems
Here are four technologies that may have a profound effect on how we eat in the future:
1. Automated Vertical Farms
It’s already clear that vertical farming is incredibly effective. By stacking farms on top of another and using automation, vertical farms can produce 100x more effectively per acre than conventional agricultural techniques.
They grow crops at twice the speed as usual, while using 40% less power, having 80% less food waste, and using 99% less water than outdoor fields. However, the problem for vertical farms is still cost – and it is not clear when they will be viable on a commercial basis.
2. Aquaponics
Another technology that has promise for the future of food is a unique combination of fish farming (aquaculture) with hydroponics.
In short, fish convert their food into nutrients that plants can absorb, while the plants clean the water for the fish. Compared to conventional farming, this technology uses about half of the water, while increasing the yield of the crops grown. As a bonus, it also can raise a significant amount of fish.
3. In Vitro Meats
Meat is costly and extremely resource intensive to produce. As just one example, to produce one pound of beef, it takes 1,847 gallons of water.
In vitro meats are one way to solve this. These self-replicating muscle tissue cultures are grown and fed nutrients in a broth, and bypass the need for having living animals altogether. Interestingly enough, market demand seems to be there: one recent study found that 70.6% of consumers are interested in trying lab grown beef.
4. Artificial Animal Products
One other route to get artificial meat is to use machine learning to grasp the complex chemistry and textures behind these products, and to find ways to replicate them. This has already been done for mayonnaise – and it’s in the works for eggs, milk, and cheese as well.
Tasting the Future of Food
As these new technologies scale and hit markets, the future of food could change drastically. Many products will flop, but others will take a firm hold in our supply chains and become culturally acceptable and commercially viable. Certainly, food will be grown locally in massive skyscrapers, and there will be decent alternatives to be found for both meat or animal products in the market.
With the global population rising by more than a million people each week, finding and testing new solutions around food will be essential to make the most out of limited resources.
Technology
All of the Grants Given by the U.S. CHIPS Act
Intel, TSMC, and more have received billions in subsidies from the U.S. CHIPS Act in 2024.
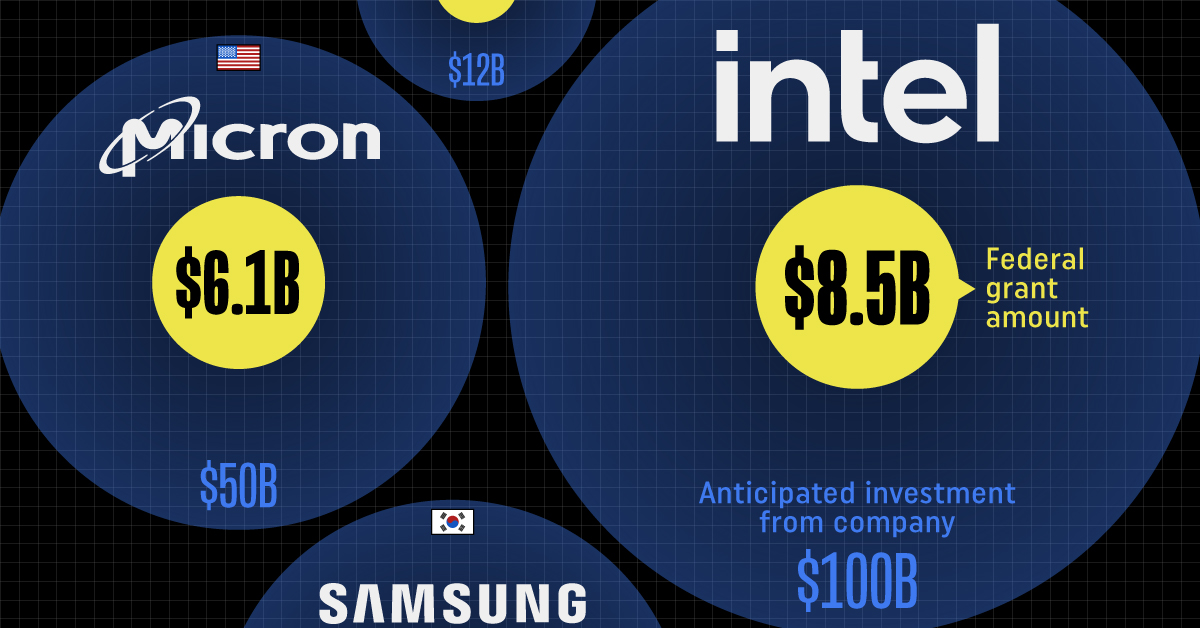
All of the Grants Given by the U.S. CHIPS Act
This was originally posted on our Voronoi app. Download the app for free on iOS or Android and discover incredible data-driven charts from a variety of trusted sources.
This visualization shows which companies are receiving grants from the U.S. CHIPS Act, as of April 25, 2024. The CHIPS Act is a federal statute signed into law by President Joe Biden that authorizes $280 billion in new funding to boost domestic research and manufacturing of semiconductors.
The grant amounts visualized in this graphic are intended to accelerate the production of semiconductor fabrication plants (fabs) across the United States.
Data and Company Highlights
The figures we used to create this graphic were collected from a variety of public news sources. The Semiconductor Industry Association (SIA) also maintains a tracker for CHIPS Act recipients, though at the time of writing it does not have the latest details for Micron.
Company | Federal Grant Amount | Anticipated Investment From Company |
---|---|---|
🇺🇸 Intel | $8,500,000,000 | $100,000,000,000 |
🇹🇼 TSMC | $6,600,000,000 | $65,000,000,000 |
🇰🇷 Samsung | $6,400,000,000 | $45,000,000,000 |
🇺🇸 Micron | $6,100,000,000 | $50,000,000,000 |
🇺🇸 GlobalFoundries | $1,500,000,000 | $12,000,000,000 |
🇺🇸 Microchip | $162,000,000 | N/A |
🇬🇧 BAE Systems | $35,000,000 | N/A |
BAE Systems was not included in the graphic due to size limitations
Intel’s Massive Plans
Intel is receiving the largest share of the pie, with $8.5 billion in grants (plus an additional $11 billion in government loans). This grant accounts for 22% of the CHIPS Act’s total subsidies for chip production.
From Intel’s side, the company is expected to invest $100 billion to construct new fabs in Arizona and Ohio, while modernizing and/or expanding existing fabs in Oregon and New Mexico. Intel could also claim another $25 billion in credits through the U.S. Treasury Department’s Investment Tax Credit.
TSMC Expands its U.S. Presence
TSMC, the world’s largest semiconductor foundry company, is receiving a hefty $6.6 billion to construct a new chip plant with three fabs in Arizona. The Taiwanese chipmaker is expected to invest $65 billion into the project.
The plant’s first fab will be up and running in the first half of 2025, leveraging 4 nm (nanometer) technology. According to TrendForce, the other fabs will produce chips on more advanced 3 nm and 2 nm processes.
The Latest Grant Goes to Micron
Micron, the only U.S.-based manufacturer of memory chips, is set to receive $6.1 billion in grants to support its plans of investing $50 billion through 2030. This investment will be used to construct new fabs in Idaho and New York.
-
Education1 week ago
How Hard Is It to Get Into an Ivy League School?
-
Technology2 weeks ago
Ranked: Semiconductor Companies by Industry Revenue Share
-
Markets2 weeks ago
Ranked: The World’s Top Flight Routes, by Revenue
-
Demographics2 weeks ago
Population Projections: The World’s 6 Largest Countries in 2075
-
Markets2 weeks ago
The Top 10 States by Real GDP Growth in 2023
-
Demographics2 weeks ago
The Smallest Gender Wage Gaps in OECD Countries
-
Economy2 weeks ago
Where U.S. Inflation Hit the Hardest in March 2024
-
Green2 weeks ago
Top Countries By Forest Growth Since 2001