Technology
Visualizing the Unicorn Landscape in 2019
Visualizing the Unicorn Landscape in 2019
It was only six years ago that venture capitalist Aileen Lee coined the term “unicorn” to describe any privately-held startup worth $1 billion or more.
At the time, such valuations were so rare that they deserved a special name – but since then, it’s fair to say that the landscape has shifted dramatically. The startup boom intensified, and capital flowed into private companies at an unprecedented pace.
In recent times, unicorns have multiplied more like rabbits, and investors have propped up the combined value of the world’s 326 unicorns to the tune of $1.1 trillion.
Breaking down the World’s 326 Unicorns
Today’s chart uses data from the Unicorn Tracker created by CB Insights, and it breaks down the unicorn landscape by sector, valuation, and country.
Let’s start by looking at the biggest unicorns currently in existence:
Rank | Company | Valuation ($B) | Country | Sector |
---|---|---|---|---|
#1 | Toutiao (ByteDance) | $75 | China | Media |
#2 | Uber | $72 | United States | On-Demand |
#3 | Didi Chuxing | $56 | China | On-Demand |
#4 | WeWork | $47 | United States | Other |
#5 | JUUL Labs | $38 | United States | Other |
#6 | Airbnb | $29 | United States | eCommerce |
#7 | Stripe | $23 | United States | Fintech |
#8 | SpaceX | $19 | United States | Other |
#9 | Epic Games | $15 | United States | Other |
#10 | GrabTaxi | $14 | Singapore | On-Demand |
ByteDance is the world’s largest unicorn at a $75 billion valuation. The company owns Toutiao, a popular machine-learning enabled content platform in China that customizes feeds based on a user’s reading preferences. It also owns video sharing platform Tik Tok.
Experts are estimating that over 100 unicorns could IPO in 2019, including Uber and Airbnb from the above list.
So far this year, Lyft and Pinterest have already hit the public market – and another recent unicorn to IPO was conferencing platform Zoom Video, which has seen shares increase 120% in price since its impressive mid-April debut.
Unicorns by Sector
The two most common sectors for unicorns are Internet Software Services and E-commerce.
Sector | # of Unicorns | Valuation ($B) |
---|---|---|
Internet Software Services | 82 | $153 |
e-commerce | 44 | $129 |
Fintech | 32 | $94 |
Healthcare | 30 | $76 |
On Demand | 23 | $200 |
Hardware | 14 | $56 |
Data analytics | 12 | $27 |
Social | 11 | $27 |
Autotech | 11 | $23 |
Media | 8 | $89 |
Travel Tech | 7 | $11 |
Cybersecurity | 7 | $15 |
Other | 45 | $186 |
Total | 326 | $1,086 billion |
However, as you can see, the segment most valued by investors is On-Demand, which includes companies like Uber, Didi Chuxing, and DoorDash.
Unicorns by Geography
Nearly half of the world’s unicorns come from the U.S., but China also has an impressive roster of highly valued startups.
Country | # of Unicorns | % |
---|---|---|
USA | 156 | 47.9% |
China | 94 | 28.8% |
UK | 17 | 5.2% |
India | 13 | 4.0% |
Germany | 8 | 2.5% |
South Korea | 6 | 1.8% |
Rest of World | 32 | 9.8% |
Total | 326 |
Strangely, outside of the six major countries listed above, the rest of the world only combines for a measly 32 unicorns – less than 10% of the global total.
Unicorns by Valuation
Seven unicorns – including Uber, WeWork, Airbnb, and ByteDance – account for almost 30% of all of the value of the entire landscape.
Valuation Range | # of Unicorns | Value ($B) | % of Value |
---|---|---|---|
$20+ billion | 7 | $321 | 29.5% |
$10-20 billion | 13 | $151 | 13.9% |
$5-10 billion | 26 | $153 | 14.1% |
$1-5 billion | 280 | $461 | 42.5% |
Total | 326 | $1,086 |
The bottom of the pyramid ($1-5 billion in valuation) holds 280 companies. Added together, they are worth $461 billion, which is equal to 42.5% of the unicorn total.
Technology
All of the Grants Given by the U.S. CHIPS Act
Intel, TSMC, and more have received billions in subsidies from the U.S. CHIPS Act in 2024.
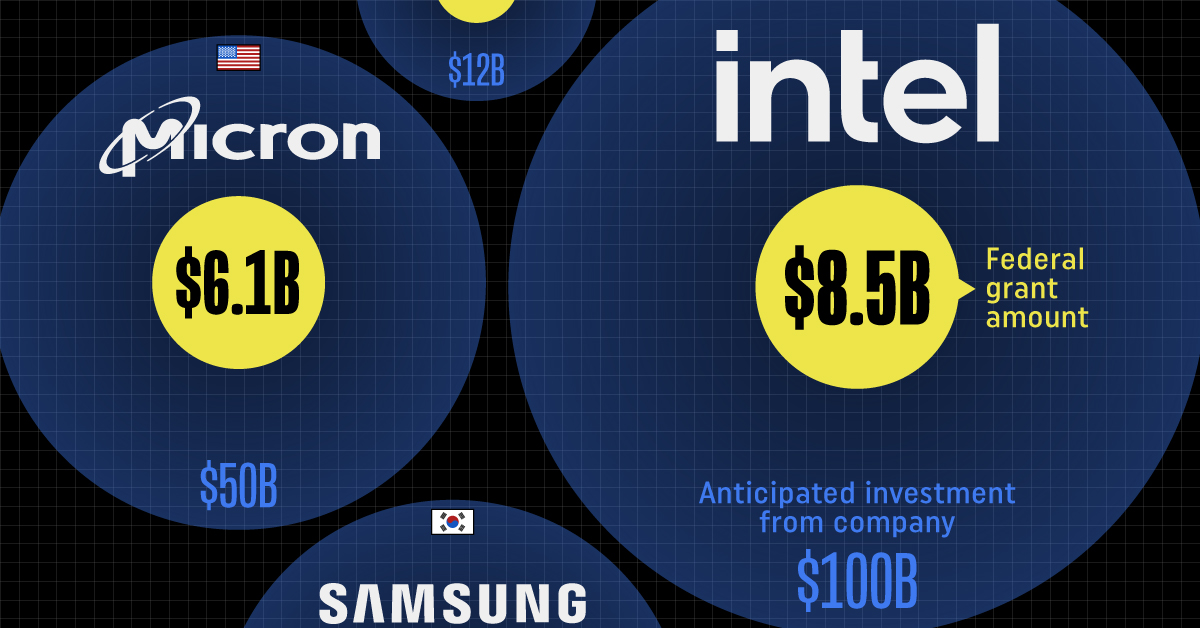
All of the Grants Given by the U.S. CHIPS Act
This was originally posted on our Voronoi app. Download the app for free on iOS or Android and discover incredible data-driven charts from a variety of trusted sources.
This visualization shows which companies are receiving grants from the U.S. CHIPS Act, as of April 25, 2024. The CHIPS Act is a federal statute signed into law by President Joe Biden that authorizes $280 billion in new funding to boost domestic research and manufacturing of semiconductors.
The grant amounts visualized in this graphic are intended to accelerate the production of semiconductor fabrication plants (fabs) across the United States.
Data and Company Highlights
The figures we used to create this graphic were collected from a variety of public news sources. The Semiconductor Industry Association (SIA) also maintains a tracker for CHIPS Act recipients, though at the time of writing it does not have the latest details for Micron.
Company | Federal Grant Amount | Anticipated Investment From Company |
---|---|---|
🇺🇸 Intel | $8,500,000,000 | $100,000,000,000 |
🇹🇼 TSMC | $6,600,000,000 | $65,000,000,000 |
🇰🇷 Samsung | $6,400,000,000 | $45,000,000,000 |
🇺🇸 Micron | $6,100,000,000 | $50,000,000,000 |
🇺🇸 GlobalFoundries | $1,500,000,000 | $12,000,000,000 |
🇺🇸 Microchip | $162,000,000 | N/A |
🇬🇧 BAE Systems | $35,000,000 | N/A |
BAE Systems was not included in the graphic due to size limitations
Intel’s Massive Plans
Intel is receiving the largest share of the pie, with $8.5 billion in grants (plus an additional $11 billion in government loans). This grant accounts for 22% of the CHIPS Act’s total subsidies for chip production.
From Intel’s side, the company is expected to invest $100 billion to construct new fabs in Arizona and Ohio, while modernizing and/or expanding existing fabs in Oregon and New Mexico. Intel could also claim another $25 billion in credits through the U.S. Treasury Department’s Investment Tax Credit.
TSMC Expands its U.S. Presence
TSMC, the world’s largest semiconductor foundry company, is receiving a hefty $6.6 billion to construct a new chip plant with three fabs in Arizona. The Taiwanese chipmaker is expected to invest $65 billion into the project.
The plant’s first fab will be up and running in the first half of 2025, leveraging 4 nm (nanometer) technology. According to TrendForce, the other fabs will produce chips on more advanced 3 nm and 2 nm processes.
The Latest Grant Goes to Micron
Micron, the only U.S.-based manufacturer of memory chips, is set to receive $6.1 billion in grants to support its plans of investing $50 billion through 2030. This investment will be used to construct new fabs in Idaho and New York.
-
Debt1 week ago
How Debt-to-GDP Ratios Have Changed Since 2000
-
Markets2 weeks ago
Ranked: The World’s Top Flight Routes, by Revenue
-
Countries2 weeks ago
Population Projections: The World’s 6 Largest Countries in 2075
-
Markets2 weeks ago
The Top 10 States by Real GDP Growth in 2023
-
Demographics2 weeks ago
The Smallest Gender Wage Gaps in OECD Countries
-
United States2 weeks ago
Where U.S. Inflation Hit the Hardest in March 2024
-
Green2 weeks ago
Top Countries By Forest Growth Since 2001
-
United States2 weeks ago
Ranked: The Largest U.S. Corporations by Number of Employees