Technology
The Game of Life: Visualizing China’s Social Credit System
The Game of Life: Visualizing China’s Social Credit System
In an attempt to imbue trust, China has announced a plan to implement a national ranking system for its citizens and companies. Currently in pilot mode, the new system will be rolled out in 2020, and go through numerous iterations before becoming official.
While the system may be a useful tool for China to manage its growing 1.4 billion population, it has triggered global concerns around the ethics of big data, and whether the system is a breach of fundamental human rights.
Today’s infographic looks at how China’s proposed social credit system could work, and what the implications might be.
The Government is Always Watching
Currently, the pilot system varies from place to place, whereas the new system is envisioned as a unified system. Although the pilot program may be more of an experiment than a precursor, it gives a good indication of what to expect.
In the pilot system, each citizen is assigned 1,000 points and is consistently monitored and rated on how they behave. Points are earned through good deeds, and lost for bad behavior. Users increase points by donating blood or money, praising the government on social media, and helping the poor. Rewards for such behavior can range from getting a promotion at work fast-tracked, to receiving priority status for children’s school admissions.
In contrast, not visiting one’s aging parents regularly, spreading rumors on the internet, and cheating in online games are considered antisocial behaviors. Punishments include public shaming, exclusion from booking flights or train tickets, and restricted access to public services.
Big Data Goes Right to the Source
The perpetual surveillance that comes with the new system is expected to draw on huge amounts of data from a variety of traditional and digital sources.
Police officers have used AI-powered smart glasses and drones to effectively monitor citizens. Footage from these devices showing antisocial behavior can be broadcast to the public to shame the offenders, and deter others from behaving similarly.
For more serious offenders, some cities in China force people to repay debts by switching the person’s ringtone without their permission. The ringtone begins with the sound of a police siren, followed by a message such as:
“The person you are calling has been listed as a discredited person by the local court. Please urge this person to fulfill his or her legal obligations.”
Two of the largest companies in China, Tencent and Alibaba, were enlisted by the People’s Bank of China to play an important role in the credit system, raising the issue of third-party data security. WeChat—China’s largest social media platform, owned by Tencent—tracked behavior and ranked users accordingly, while displaying their location in real-time.
Following data concerns, these tech companies—and six others—were not awarded any licenses by the government. However, social media giants are still involved in orchestrating the public shaming of citizens who misbehave.
The Digital Dang’an
The social credit system may not be an entirely new initiative in China. The dang’an (English: record) is a paper file containing an individual’s school reports, information on physical characteristics, employment records, and photographs.
These dossiers, which were first used in the Maoist years, helped the government in maintaining control of its citizens. This gathering of citizen’s data for China’s social credit system may in fact be seen as a revival of the principle of dang’an in the digital era, with the system providing a powerful tool to monitor citizens whose data is more difficult to capture.
Is the System Working?
In 2018, people with a low score were prohibited from buying plane tickets almost 18 million times, while high-speed train ticket transactions were blocked 5.5 million times. A further 128 people were prohibited from leaving China, due to unpaid taxes.
The system could have major implications for foreign business practices—as preference could be given to companies already ranked in the system. Companies with higher scores will be rewarded with incentives which include lower tax rates and better credit conditions, with their behavior being judged in areas such as:
- Paid taxes
- Customs regulation
- Environmental protection
Despite the complexities of gathering vast amounts of data, the system is certainly making an impact. While there are benefits to having a standardized scoring system, and encouraging positive behavior—will it be worth the social cost of gamifying human life?
Technology
All of the Grants Given by the U.S. CHIPS Act
Intel, TSMC, and more have received billions in subsidies from the U.S. CHIPS Act in 2024.
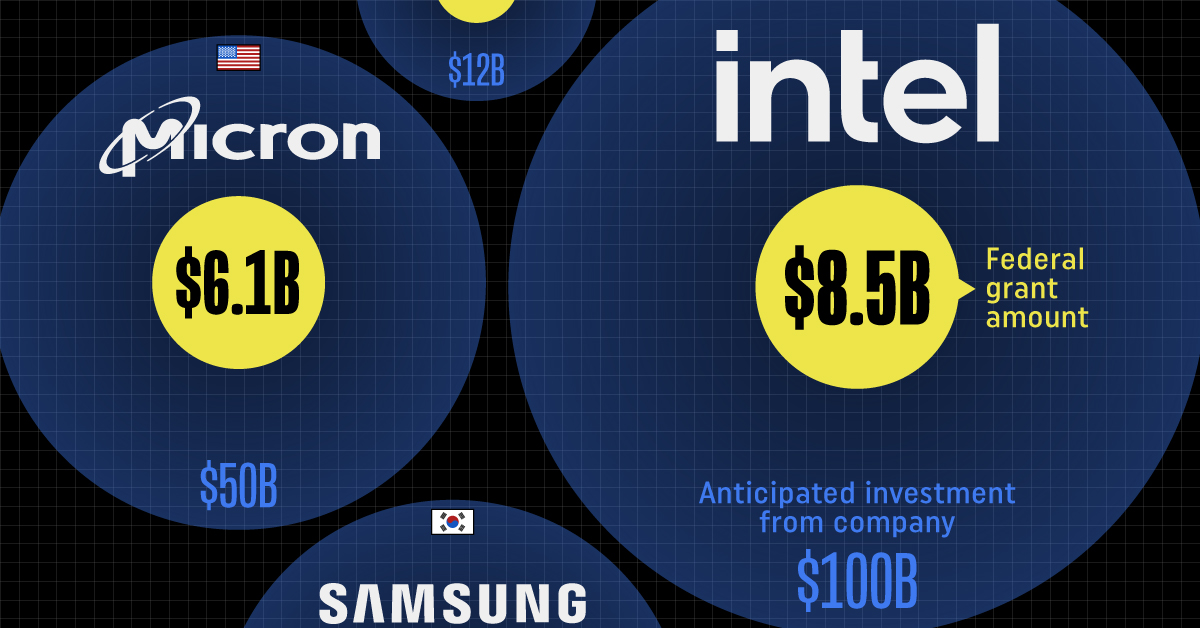
All of the Grants Given by the U.S. CHIPS Act
This was originally posted on our Voronoi app. Download the app for free on iOS or Android and discover incredible data-driven charts from a variety of trusted sources.
This visualization shows which companies are receiving grants from the U.S. CHIPS Act, as of April 25, 2024. The CHIPS Act is a federal statute signed into law by President Joe Biden that authorizes $280 billion in new funding to boost domestic research and manufacturing of semiconductors.
The grant amounts visualized in this graphic are intended to accelerate the production of semiconductor fabrication plants (fabs) across the United States.
Data and Company Highlights
The figures we used to create this graphic were collected from a variety of public news sources. The Semiconductor Industry Association (SIA) also maintains a tracker for CHIPS Act recipients, though at the time of writing it does not have the latest details for Micron.
Company | Federal Grant Amount | Anticipated Investment From Company |
---|---|---|
🇺🇸 Intel | $8,500,000,000 | $100,000,000,000 |
🇹🇼 TSMC | $6,600,000,000 | $65,000,000,000 |
🇰🇷 Samsung | $6,400,000,000 | $45,000,000,000 |
🇺🇸 Micron | $6,100,000,000 | $50,000,000,000 |
🇺🇸 GlobalFoundries | $1,500,000,000 | $12,000,000,000 |
🇺🇸 Microchip | $162,000,000 | N/A |
🇬🇧 BAE Systems | $35,000,000 | N/A |
BAE Systems was not included in the graphic due to size limitations
Intel’s Massive Plans
Intel is receiving the largest share of the pie, with $8.5 billion in grants (plus an additional $11 billion in government loans). This grant accounts for 22% of the CHIPS Act’s total subsidies for chip production.
From Intel’s side, the company is expected to invest $100 billion to construct new fabs in Arizona and Ohio, while modernizing and/or expanding existing fabs in Oregon and New Mexico. Intel could also claim another $25 billion in credits through the U.S. Treasury Department’s Investment Tax Credit.
TSMC Expands its U.S. Presence
TSMC, the world’s largest semiconductor foundry company, is receiving a hefty $6.6 billion to construct a new chip plant with three fabs in Arizona. The Taiwanese chipmaker is expected to invest $65 billion into the project.
The plant’s first fab will be up and running in the first half of 2025, leveraging 4 nm (nanometer) technology. According to TrendForce, the other fabs will produce chips on more advanced 3 nm and 2 nm processes.
The Latest Grant Goes to Micron
Micron, the only U.S.-based manufacturer of memory chips, is set to receive $6.1 billion in grants to support its plans of investing $50 billion through 2030. This investment will be used to construct new fabs in Idaho and New York.
-
Education1 week ago
How Hard Is It to Get Into an Ivy League School?
-
Technology2 weeks ago
Ranked: Semiconductor Companies by Industry Revenue Share
-
Markets2 weeks ago
Ranked: The World’s Top Flight Routes, by Revenue
-
Demographics2 weeks ago
Population Projections: The World’s 6 Largest Countries in 2075
-
Markets2 weeks ago
The Top 10 States by Real GDP Growth in 2023
-
Demographics2 weeks ago
The Smallest Gender Wage Gaps in OECD Countries
-
Economy2 weeks ago
Where U.S. Inflation Hit the Hardest in March 2024
-
Green2 weeks ago
Top Countries By Forest Growth Since 2001