Technology
Charted: The Exponential Growth in AI Computation
Click to view this graphic in a higher-resolution.
Charted: The Exponential Growth in AI Computation
Electronic computers had barely been around for a decade in the 1940s, before experiments with AI began. Now we have AI models that can write poetry and generate images from textual prompts. But what’s led to such exponential growth in such a short time?
This chart from Our World in Data tracks the history of AI through the amount of computation power used to train an AI model, using data from Epoch AI.
The Three Eras of AI Computation
In the 1950s, American mathematician Claude Shannon trained a robotic mouse called Theseus to navigate a maze and remember its course—the first apparent artificial learning of any kind.
Theseus was built on 40 floating point operations (FLOPs), a unit of measurement used to count the number of basic arithmetic operations (addition, subtraction, multiplication, or division) that a computer or processor can perform in one second.
Computation power, availability of training data, and algorithms are the three main ingredients to AI progress. And for the first few decades of AI advances, compute, which is the computational power needed to train an AI model, grew according to Moore’s Law.
Period | Era | Compute Doubling |
---|---|---|
1950–2010 | Pre-Deep Learning | 18–24 months |
2010–2016 | Deep Learning | 5–7 months |
2016–2022 | Large-scale models | 11 months |
Source: “Compute Trends Across Three Eras of Machine Learning” by Sevilla et. al, 2022.
However, at the start of the Deep Learning Era, heralded by AlexNet (an image recognition AI) in 2012, that doubling timeframe shortened considerably to six months, as researchers invested more in computation and processors.
With the emergence of AlphaGo in 2015—a computer program that beat a human professional Go player—researchers have identified a third era: that of the large-scale AI models whose computation needs dwarf all previous AI systems.
Predicting AI Computation Progress
Looking back at the only the last decade itself, compute has grown so tremendously it’s difficult to comprehend.
For example, the compute used to train Minerva, an AI which can solve complex math problems, is nearly 6 million times that which was used to train AlexNet 10 years ago.
Here’s a list of important AI models through history and the amount of compute used to train them.
AI | Year | FLOPs |
---|---|---|
Theseus | 1950 | 40 |
Perceptron Mark I | 1957–58 | 695,000 |
Neocognitron | 1980 | 228 million |
NetTalk | 1987 | 81 billion |
TD-Gammon | 1992 | 18 trillion |
NPLM | 2003 | 1.1 petaFLOPs |
AlexNet | 2012 | 470 petaFLOPs |
AlphaGo | 2016 | 1.9 million petaFLOPs |
GPT-3 | 2020 | 314 million petaFLOPs |
Minerva | 2022 | 2.7 billion petaFLOPs |
Note: One petaFLOP = one quadrillion FLOPs. Source: “Compute Trends Across Three Eras of Machine Learning” by Sevilla et. al, 2022.
The result of this growth in computation, along with the availability of massive data sets and better algorithms, has yielded a lot of AI progress in seemingly very little time. Now AI doesn’t just match, but also beats human performance in many areas.
It’s difficult to say if the same pace of computation growth will be maintained. Large-scale models require increasingly more compute power to train, and if computation doesn’t continue to ramp up it could slow down progress. Exhausting all the data currently available for training AI models could also impede the development and implementation of new models.
However with all the funding poured into AI recently, perhaps more breakthroughs are around the corner—like matching the computation power of the human brain.
Where Does This Data Come From?
Source: “Compute Trends Across Three Eras of Machine Learning” by Sevilla et. al, 2022.
Note: The time estimated to for computation to double can vary depending on different research attempts, including Amodei and Hernandez (2018) and Lyzhov (2021). This article is based on our source’s findings. Please see their full paper for further details. Furthermore, the authors are cognizant of the framing concerns with deeming an AI model “regular-sized” or “large-sized” and said further research is needed in the area.
Methodology: The authors of the paper used two methods to determine the amount of compute used to train AI Models: counting the number of operations and tracking GPU time. Both approaches have drawbacks, namely: a lack of transparency with training processes and severe complexity as ML models grow.

This article was published as a part of Visual Capitalist's Creator Program, which features data-driven visuals from some of our favorite Creators around the world.
Technology
All of the Grants Given by the U.S. CHIPS Act
Intel, TSMC, and more have received billions in subsidies from the U.S. CHIPS Act in 2024.
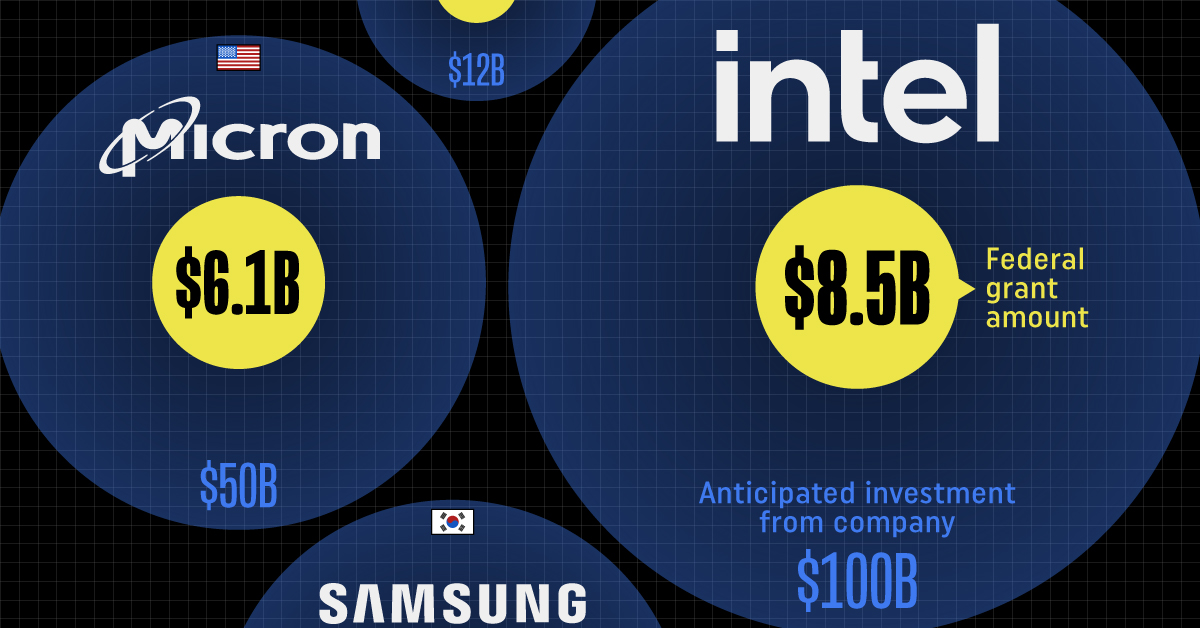
All of the Grants Given by the U.S. CHIPS Act
This was originally posted on our Voronoi app. Download the app for free on iOS or Android and discover incredible data-driven charts from a variety of trusted sources.
This visualization shows which companies are receiving grants from the U.S. CHIPS Act, as of April 25, 2024. The CHIPS Act is a federal statute signed into law by President Joe Biden that authorizes $280 billion in new funding to boost domestic research and manufacturing of semiconductors.
The grant amounts visualized in this graphic are intended to accelerate the production of semiconductor fabrication plants (fabs) across the United States.
Data and Company Highlights
The figures we used to create this graphic were collected from a variety of public news sources. The Semiconductor Industry Association (SIA) also maintains a tracker for CHIPS Act recipients, though at the time of writing it does not have the latest details for Micron.
Company | Federal Grant Amount | Anticipated Investment From Company |
---|---|---|
🇺🇸 Intel | $8,500,000,000 | $100,000,000,000 |
🇹🇼 TSMC | $6,600,000,000 | $65,000,000,000 |
🇰🇷 Samsung | $6,400,000,000 | $45,000,000,000 |
🇺🇸 Micron | $6,100,000,000 | $50,000,000,000 |
🇺🇸 GlobalFoundries | $1,500,000,000 | $12,000,000,000 |
🇺🇸 Microchip | $162,000,000 | N/A |
🇬🇧 BAE Systems | $35,000,000 | N/A |
BAE Systems was not included in the graphic due to size limitations
Intel’s Massive Plans
Intel is receiving the largest share of the pie, with $8.5 billion in grants (plus an additional $11 billion in government loans). This grant accounts for 22% of the CHIPS Act’s total subsidies for chip production.
From Intel’s side, the company is expected to invest $100 billion to construct new fabs in Arizona and Ohio, while modernizing and/or expanding existing fabs in Oregon and New Mexico. Intel could also claim another $25 billion in credits through the U.S. Treasury Department’s Investment Tax Credit.
TSMC Expands its U.S. Presence
TSMC, the world’s largest semiconductor foundry company, is receiving a hefty $6.6 billion to construct a new chip plant with three fabs in Arizona. The Taiwanese chipmaker is expected to invest $65 billion into the project.
The plant’s first fab will be up and running in the first half of 2025, leveraging 4 nm (nanometer) technology. According to TrendForce, the other fabs will produce chips on more advanced 3 nm and 2 nm processes.
The Latest Grant Goes to Micron
Micron, the only U.S.-based manufacturer of memory chips, is set to receive $6.1 billion in grants to support its plans of investing $50 billion through 2030. This investment will be used to construct new fabs in Idaho and New York.
-
Debt1 week ago
How Debt-to-GDP Ratios Have Changed Since 2000
-
Countries2 weeks ago
Population Projections: The World’s 6 Largest Countries in 2075
-
Markets2 weeks ago
The Top 10 States by Real GDP Growth in 2023
-
Demographics2 weeks ago
The Smallest Gender Wage Gaps in OECD Countries
-
United States2 weeks ago
Where U.S. Inflation Hit the Hardest in March 2024
-
Green2 weeks ago
Top Countries By Forest Growth Since 2001
-
United States2 weeks ago
Ranked: The Largest U.S. Corporations by Number of Employees
-
Maps2 weeks ago
The Largest Earthquakes in the New York Area (1970-2024)